In today’s financial markets, the pace of trading has accelerated dramatically with the rise of technological innovation. Even movements in the hum share price lse reflect how computerized systems now conduct over 70% of all trades, compared to just 30% four years ago. This transformation raises critical questions about the effects of high-frequency trading (HFT) on market stability and price movements. As trading volumes increase alongside trading frequency, regulators and investors alike question whether these developments positively or negatively impact the market. Research suggests that higher trading volumes may lead to increased stock price volatility, potentially necessitating new regulatory approaches for firms employing high-frequency strategies.
Introduction
The technological revolution has fundamentally altered how stock exchanges operate. For over a century, the New York Stock Exchange functioned as the global hub of investing, with human brokers and investors physically present on the trading floor. Today, that bustling human presence has largely disappeared, replaced by sophisticated computer systems.
High-frequency trading represents the culmination of this transformation, utilizing complex algorithms to scan multiple exchanges simultaneously. These systems analyze market conditions and historical performance patterns to anticipate price movements within milliseconds. Unlike human traders, however, these algorithms lack real understanding of the companies whose shares they trade.
The debate around HFT centers on two primary perspectives:
- Liquidity Providers: Proponents argue HFT creates essential market liquidity, enabling investors to buy and sell stocks immediately at fair prices.
- Market Manipulators: Critics contend HFT allows computers to detect and capitalize on price movements before human investors can react, potentially generating billions in profits at the expense of ordinary investors.
Literature Review
The relationship between trading volume and price changes has been studied extensively. Karpoff’s influential 1987 survey established a framework for understanding this connection, providing insights into market structure and futures market dynamics. Interestingly, this relationship has evolved significantly since those early studies, which predated the era of high-frequency trading.
More recent research by Chakravarty (2001) examined “stealth trading” in stock markets, finding that medium-sized trades produce disproportionately large cumulative price changes relative to their share of total trade volume. This suggests institutional investors, including HFT firms, exert significant influence on price movements. The effect may be amplified by algorithmic trading that triggers additional trades in the same direction, creating a multiplier effect that grows as HFT becomes more prevalent.
Key considerations from existing research include:
- The “bandwagon effect” where consensus views anticipate severe price changes, prompting overwhelming buy or sell movements
- Davidson’s (1998) recommendation for market makers willing to “lean into the wind” when markets deviate from fundamentals
- Conflicting regulatory perspectives between the EU and US versus industry representatives who warn restrictions might exacerbate market dislocations
Theoretical Model
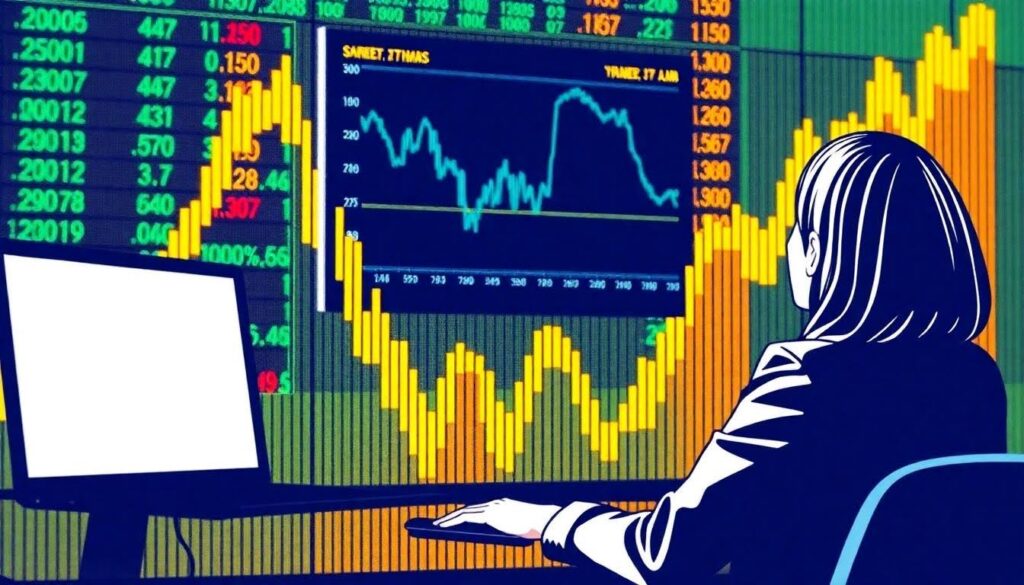
The impact of HFT on stock prices can be understood through basic supply and demand principles. In stock markets, supply remains relatively fixed or inelastic, while demand fluctuates based on intrinsic value assessments, fundamental analysis, and investor expectations.
When trading volume increases, demand shifts rightward (more buyer-initiated trades) or leftward (more seller-initiated trades), directly affecting stock prices. HFT firms, acting as informed investors, initially shift demand curves, potentially triggering bandwagon investing as other market participants observe and follow these trends.
Though HFT firms may quickly enter and exit positions—sometimes within seconds—their initial actions can spark extended demand shifts as conventional investors respond to apparent trends. This amplification effect can push prices significantly higher or lower than company fundamentals might warrant.
The core concern with this model is that computers generating most trading volume possess no understanding of the underlying companies. This disconnect creates potential for artificial inflation or deflation, where prices move substantially away from intrinsic company values based primarily on algorithm-driven trading rather than fundamental assessment.
Empirical Model
To test these theoretical relationships, we analyzed weekly data from the Dow Jones Industrial Average between January 2000 and December 2009. The DJIA was selected as an index of 30 blue-chip companies, providing both stability and a reasonable representation of overall market conditions.
Five primary variables were recorded:
- Opening price
- Closing price
- Weekly high and low prices
- Average daily trading volume
Two additional variables were derived:
- POC (absolute difference between open and close prices)
- PHL (absolute difference between high and low prices during the week)
The methodology required several preparatory steps. First, volume data was transformed into growth rates to focus on relative changes rather than absolute values. Next, stationarity test s— Augmented Dickey Fuller (ADF) and Kwaitowski, Phillips, Schmidt, and Shin (KPSS)—were applied to ensure valid time series analysis.
Once stationarity was confirmed, ordinary least squares regressions examined the relationship between volume changes and price volatility, using the following general equations:
- PHL = c + βΔVolume (measuring impact on high-low price differences)
- POC = c + βΔVolume (measuring impact on open-close price differences)
Results
The analysis revealed significant changes in trading patterns over the decade studied. From 2000-2004, average weekly trading volume showed modest growth, but from 2004-2009, coinciding with HFT expansion, volume increased exponentially. This dramatic growth aligns with the technological advancements enabling faster, larger trades.
Unit root and stationarity tests confirmed that while original volume data contained unit roots and lacked stationarity, first-order differences achieved stationarity required for regression analysis. The price variables (POC and PHL) demonstrated stationarity in levels.
Variable | R-squared | Coefficient | Probability |
PHL | 0.605 | 0.777 | 0.000 |
POC | 0.029 | 0.897 | 0.001 |
The regression results yielded an important distinction between the two price volatility measures. For PHL (high-low price differences), the model showed a strong relationship with volume changes, with an R-squared of 0.605 and a coefficient of 0.777—indicating that a 10% increase in trading volume corresponds to a 7.77% increase in high-low price volatility. This relationship was statistically significant at the 99% confidence level.
Conversely, the relationship between volume changes and POC (open-close price differences) was much weaker. Though statistically significant, the low R-squared value (0.029) suggests limited practical relationship between trading volume and weekly open-close price changes.
Conclusion
These findings offer nuanced insights into how trading frequency affects stock prices. The strong relationship between volume and intraweek price volatility (PHL) confirms that increased trading activity, particularly from HFT, creates substantial price movements during trading periods. However, the weak relationship with open-close price differences (POC) suggests markets ultimately correct these fluctuations by week’s end.
For investors, the implications of these findings include:
- HFT creates significant short-term volatility that affects market sentiment and potentially trading psychology
- Despite intra-week volatility, markets appear to self-correct over longer timeframes
- Weekly closing price stability suggests limited long-term impact from high-frequency trading
From a policy perspective, these results suggest that restrictions on trading volume alone may not be necessary to maintain market integrity. While HFT creates intraweek volatility, its long-term impact appears minimal based on weekly closing price stability.
Future research could extend this analysis by examining more granular timeframes—possibly daily or minute-by-minute data—to uncover volatility patterns that weekly analysis might obscure. Additionally, sector-specific studies could determine whether certain industries experience more pronounced effects from trading frequency variations than others.